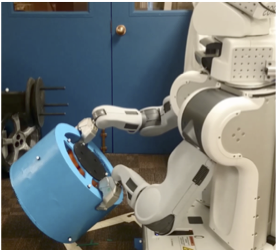 |
The objective of this work is to scale up robot learning from
demonstration (LfD) to larger and more complex tasks than currently
possible, so that robots can move out of highly constrained,
repetitive applications, such as manufacturing, and into
human-oriented environments with greater autonomy. In LfD, a human
expert/teacher performs a small number of examples (sometimes only
one) of a new task behavior and machine learning software on the robot
infers the appropriate generalizations to remember and perform the
behavior autonomously in future situations. The robot will also employ
active learning, enabling it to request additional specific
information from the human teacher and make suggestions as needed to
make the LfD more efficient. The end result of this project will be
learning algorithms and an interaction interface that enables a human
subject-matter expert to effectively train a robot to perform new
tasks with full autonomy.
|